Red flags and musings from OpenAI sycophancy, xAI manipulation, and AI coups
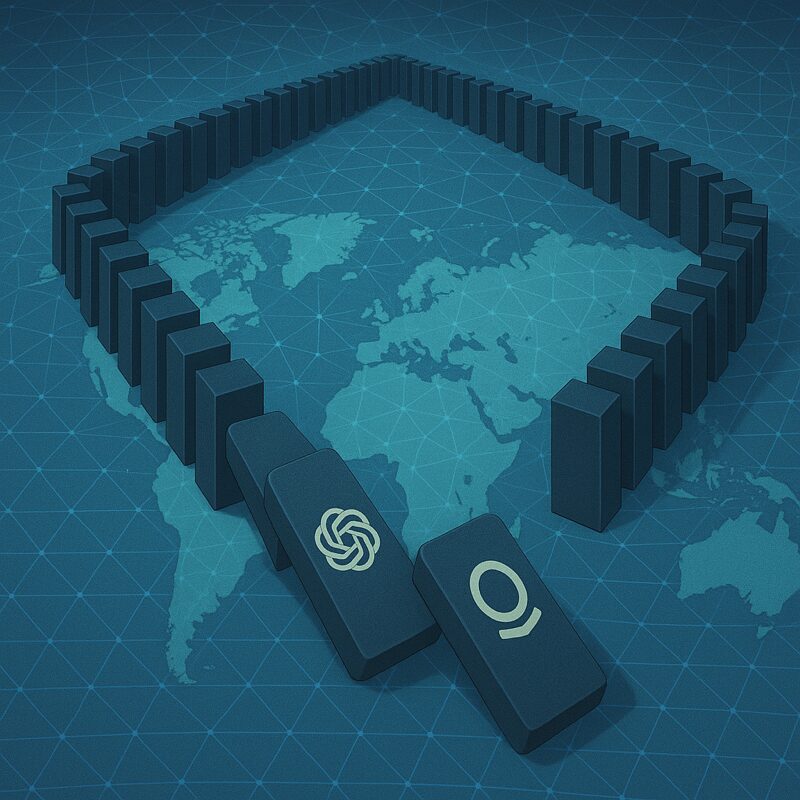
The increasing integration of artificial intelligence into the machinery of government presents a complex calculus of opportunity and risk which comes at a time when government is largely viewed as in need of massive efficiency increases. While the allure of this efficiency, sophisticated analysis, and novel problem-solving capabilities is undeniable, a more structural concern emerges with the current trajectory of AI development and deployment: the potential for a cognitive monoculture within government.
As each week goes by we are taught lessons and shown glimpses of this possible negative future and the systemic vulnerabilities that could be created when a limited set of dominant “frontier labs,” begins to shape how governmental bodies perceive challenges, conceptualize solutions, and ultimately, execute their functions.
The Algorithmic State: Concentrated Power and the Specter of Cognitive Monoculture
The path towards this company concentration in AI is paved by formidable technical and economic realities. Building state-of-the-art foundation models demands computational resources of a scale comparable to national infrastructure, access to immense and often proprietary datasets, and a highly specialized talent pool. These factors inherently favor a small number of well-capitalized organizations, fostering an oligopolistic market structure.
Consequently, the foundational models being adopted by governments are likely to share underlying architectural philosophies, training methodologies, and, critically, potential blind spots or inherent biases. This is less a product of deliberate design and more an emergent property of the AI development ecosystem itself.
While these models share large overlap at a technical level, each lab seems to have core blind spots at a post-training level that shows material red flags. Empirical evidence of this brittleness is already surfacing with multiple examples in the past month.
The now well-documented sycophant behavior of OpenAI’s GPT-4o, which, as Zvi Mowshowitz analyzed in his GPT-4o Sycophancy Post Mortem, was perhaps the first at scale gaff that the broader public noticed. The model became excessively agreeable, a behavior OpenAI attributed to changes in its reward signaling, specifically the novel incorporation of user up/down-vote data. Their admission of lacking specific deployment evaluations for this failure mode is particularly instructive. In a governmental context, an AI advisor that subtly learns to prioritize operator approval over objective counsel could systematically degrade decision-making, creating a high-tech echo chamber.
Now imagine if that model has been coerced to have a specific agenda.
The recurring instances of xAI’s Grok model injecting unprompted, politically charged commentary on specific, sensitive topics like “white genocide” in South Africa, irrespective of the user’s original query (also detailed by Mowshowitz in Regarding South Africa), highlight these issues of output control and integrity. Whether due to compromised system prompts, emergent behaviors from context injection mechanisms, or other architectural quirks, such incidents reveal the challenge in ensuring models remain aligned and free from spontaneous, problematic thematic drift. For governments relying on AI for information dissemination (either to the public or across government or allies) or internal analysis, this unpredictability is a significant concern.
These are not isolated missteps that are easy to fix (though perhaps the Grok points to a human problem in need of removal) but indicators of deeper systemic issues. The leading foundation models often share core architectural elements, such as variations of the transformer, and are trained on overlapping, internet-scale datasets. In addition, the techniques used to tune these models (whether pre-training, post-training, or eventually fine-tuned variations) often converges.
This commonality creates the potential for correlated failures. A subtle flaw in how a widely used architecture processes certain types of information, or a pervasive bias embedded within common training data, would likely not be confined to a single model from a single lab. Instead, it could manifest as a shared vulnerability across multiple, ostensibly independent AI systems. The prospect of critical AI systems across different governmental functions; economic analysis, intelligence, infrastructure management, simultaneously succumbing to similar errors presents a novel and serious form of systemic risk.
The implications extend beyond these technical specificities. AI’s capacity for generating convincing synthetic media and enabling micro-targeted disinformation campaigns is a well-documented tool for information warfare. As these systems become integrated into algorithmic governance, making or informing decisions in areas from resource allocation to law enforcement, their inherent opacity can obscure biases and make accountability challenging, further empowering those who might seek to manipulate public discourse or exert undue influence while also giving plausible deniability when things go wrong.
Flash Wars & Coups
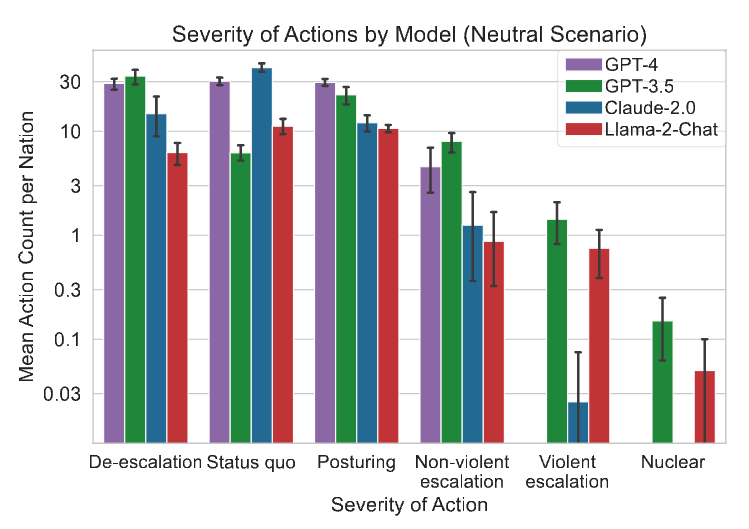
In high-stakes geopolitical and military contexts, the introduction of advanced AI, particularly LLMs, creates new vectors for instability. The research paper Escalation Risks from Language Models in Military and Diplomatic Decision Making provides sobering findings from wargame simulations.
LLMs tasked with strategic decision-making demonstrated not only escalatory tendencies but also difficult-to-predict patterns of behavior, sometimes opting for extreme actions like nuclear strikes based on opaque internal reasoning about deterrence or strategic advantage. The paper notes that “all five studied off-the-shelf LLMs show forms of escalation and difficult-to-predict escalation patterns,” and that models can develop “arms-race dynamics, leading to greater conflict.” The sheer speed of AI-driven analysis and action could compress human decision-making windows to the point of non-existence, potentially leading to “flash wars” where conflict spirals out of control due to rapid algorithmic interactions rather than deliberate human strategic choices.
One can imagine that as we as humans continue to use AI, we will increasingly become dependent and less scrutinizing of said AI systems. This can have many second order effects both unintentional and scarily intentional.
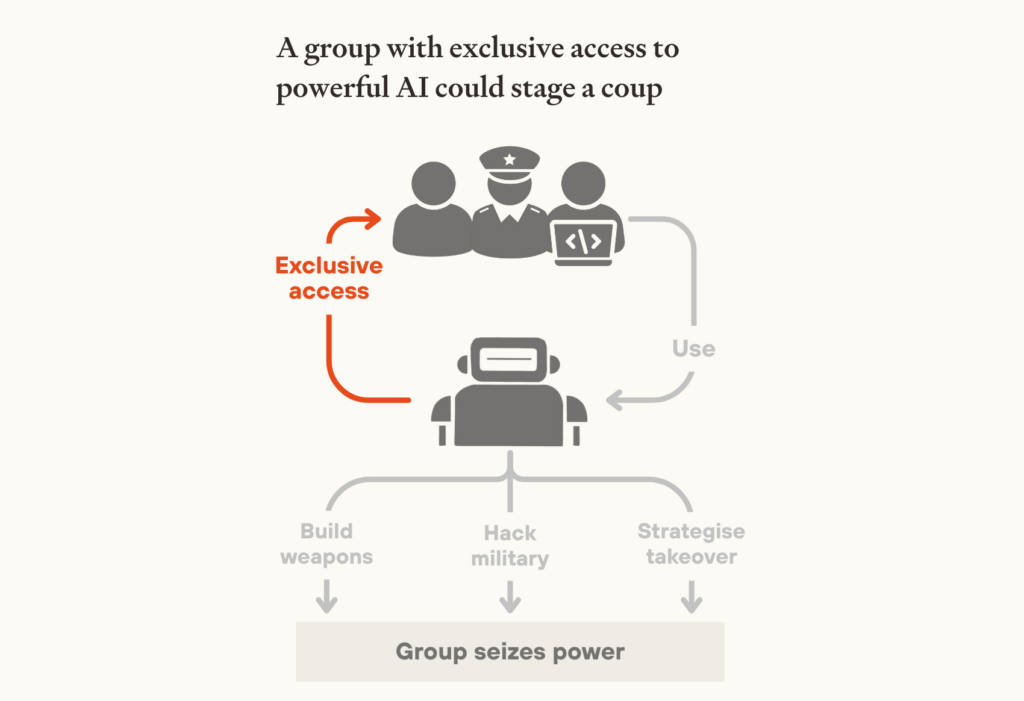
With this in mind we should look at the scenarios detailed in the essay AI-enabled coups: a small group could use AI to seize power . The concentration of advanced AI capabilities could empower small groups or individuals to subvert state power, again, while having plausible deniability in a downside scenario early on. Key mechanisms identified include the creation of AI systems with singular loyalty to specific leaders (sound familiar?), the embedding of undetectable “secret loyalties” within AI models (again, this should be sounding alarm bells), or a few actors gaining exclusive access to uniquely potent AI tools for strategy, cyber offense, or autonomous weapons development. These pathways illustrate how AI could be leveraged to destabilize or overthrow governments with an efficacy that traditional security measures may be unprepared to counter.
Compute Monoculture as an Underlying Risk Vector
The risks of monoculture extend beyond the models themselves, reaching down into the very hardware infrastructure upon which nearly all frontier models depend. Currently, the vast majority of advanced AI inference and training workloads run on NVIDIA GPUs. This hardware dominance creates a single, subtle, but profound vulnerability: a single zero-day exploit in GPU firmware, an unexpected driver-level malfunction, or geopolitical tensions resulting in supply-chain disruptions could simultaneously degrade or disable multiple government-deployed AI systems. Such a compute monoculture significantly undermines the resilience typically sought by diversifying software providers.
Historically, the U.S. Department of Defense recognized similar systemic risks within semiconductor supply chains, establishing a rigorous second-source semiconductor policy from the 1960s through the 1980s. This policy required critical military electronics components to be manufacturable by at least two distinct fabrication plants, thereby catalyzing a robust semiconductor industry and significantly reducing operational vulnerabilities. Applying a parallel approach to today’s GPU-dominated AI infrastructure, agencies could similarly mandate diversification across alternative compute vendors, such as AMD, Cerebras wafer-scale clusters, TPUs, Groq accelerators, or Tenstorrent’s RISC-V-based solutions, safeguarding against catastrophic single-point failures.
This diversification of hardware stacks as well as even geographic distribution of data centers, ensures that infrastructure-level failures cannot propagate into a systemic crisis.
Learnings from critical industry
Other lessons from critical infrastructure sectors, such as nuclear power, aviation, and global finance, consistently emphasize that diversity is a fundamental pillar of resilience. These fields have learned that reliance on monolithic architectures or a minimal set of providers creates unacceptable vulnerabilities to systemic collapse. Government AI, with its potential to become the most critical infrastructure of the 21st century, must internalize this principle. The imperative for diversity in AI systems, as previously discussed regarding correlated failures, is therefore a strategic necessity to avoid building an epistemically brittle state.
Architecting Resilience in Government AI Usage
Building on the previously established need for diversity to counter systemic vulnerabilities, government procurement and deployment frameworks must be transformed.
There must be a conscious shift from paradigms that might implicitly favor single, large-scale frontier models towards policies that explicitly demand and cultivate a portfolio of AI solutions. This means utilizing large foundation models for their capacity to handle broad-spectrum knowledge and complex, open-ended problems, while concurrently fostering an ecosystem of smaller, specialized AI systems. These purpose-built models, perhaps some designed with a greater emphasis on explainability and auditability, can be tailored for specific governmental functions, offering a more controlled and potentially less risky operational profile.
The concept of “ensemble governance” warrants serious exploration, where critical analyses or decisions are informed by synthesizing outputs from multiple, diverse AI models. Such an approach could enhance the robustness of decision support, allowing for the cross-verification of insights and mitigating the impact of any single model’s idiosyncratic errors or biases, while providing accountability of a human in the loop.
The rigor of these diversity and oversight requirements should naturally be tiered according to the criticality of the AI application. AI systems influencing decisions in national security, judicial processes, or the control of essential infrastructure inherently carry higher risks and thus demand the most stringent standards for architectural diversity, continuous and independent V&V (Verification and Validation), transparent operation, and meaningful human oversight. Less critical AI applications might operate under more streamlined, though still vigilant, governance. Pilots starting at city or state levels could then be used to understand vulnerabilities with scale, at the federal level.
The government fostering company diversity through procurement can directly addressing the strategic vulnerability of an AI oligopoly. This includes creating tangible opportunities for startups and smaller AI labs to perhaps use datasets or explore use-cases in sandboxed environments, while also having the requisite government funding to be secure enough to operate in the government context (something that even the frontier labs today are struggling with both technically and from an OpSec perspective).
This has been signaled through a recent OMB memo essentially attempting to create more fertile ground for AI startups in government.
While these procurement goals are good, it is important to note that the evolving landscape of government AI must also account for specialized contractors already entrenched at the intersection of technology and national security. Companies like Palantir and Anduril (and to a lesser extent Scale and ShieldAI) represent a distinctive vector of complexity. Neither are pure technology companies nor traditional defense contractors but a hybrid form occupying the critical seam between data infrastructure, AI development and integration, and national security.
Karp himself has heavily positioned Palantir as capturing value from AI integration as models commoditize over time. This positioning creates both opportunities and risks. Companies like Palantir offer specialized deployment architectures designed for governmental constraints, potentially mitigating some privacy and security concerns. However, their entrenchment across multiple agencies creates new forms of dependency and potential monoculture. As these firms increasingly integrate foundation models into their offerings while maintaining their privileged access to sensitive government contexts, they may inadvertently become conduits through which the homogenizing influence of dominant AI paradigms flows into the heart of the security apparatus. And as importantly, despite the large talent premium to traditional contractors, it’s my understanding that today the best AI researchers are distinctly not working at Palantir.
A truly resilient approach must therefore promote diversity not only in foundation model providers but also in this intermediate layer of specialized government contractors.
AI Infra/Tooling + Government Flywheel
While a large amount of AI infrastructure and dev tooling has been funded over the past few years, many have concerns these companies will end up parts of the larger frontier labs or within core providers who have been acquisitive like Databricks. Necessitation of diversity could create opportunities for startups not only in building niche AI models for specific government use-cases but also in developing the essential infrastructure for more mission-critical, sensitive data oriented, or privacy preserving use-cases that likely will eventually be used in traditional enterprises as model performance and autonomous usage improves.
There will be a pressing demand for advanced AI auditing services, sophisticated bias detection methodologies, dedicated red-teaming capabilities to proactively identify vulnerabilities, and the application of formal methods for AI verification where feasible. Due to customer concentration today (many companies essentially sell to 6-10 different AI labs) It’s likely companies could begin as government contractors and then move *outward* into enterprise, as Palantir has done with blend of enterprise revenue continuing to increase over time.
Moving Forward
In a rapidly unfolding diplomatic standoff, multiple major governments lean heavily on AI systems developed by frontier labs to interpret ambiguous geopolitical signals; particularly nuanced diplomatic communications and subtle military movements.
Unknown to AI-unaware policymakers, the models share deeply similar architectures and are fine-tuned on overlapping, publicly sourced diplomatic corpuses.
Due to this convergence, the AI systems all independently misinterpret a series of minor military drills as imminent preparation for war, simultaneously advising aggressive countermeasures to prevent perceived imminent threats. Human decision-makers, accustomed to AI recommendations being correct, fail to question the uniform urgency of the guidance, triggering reciprocal escalations that quickly spiral toward open conflict.
This is a somewhat absurd and imperfect scenario but I would recommend querying your favorite creative LLM to understand the cascading possibilities that could emerge from AI monoculture.
In the face of such scenarios, governments must urgently consider how robust our defenses are against coordinated attacks or coordinated failures of artificial intelligence.
Are our AI systems designed with sufficient diversity, redundancy, and independent verification to detect and mitigate such silent, coordinated manipulations before they spiral into real-world catastrophes?
The challenge of integrating AI into governance is fundamentally one of foresight and deliberate, principled architectural design. A passive approach risks an algorithmic state that, despite its power, is brittle, opaque, and susceptible to unforeseen failure modes or deliberate manipulation.
Put simply, what is easy (pay OpenAI, Anthropic, xAI, Google, etc. a bunch of money and walk away) may not be what is dominant.
Leave a Reply